Ph.D. Candidate Aritra Ghosh receives 2021 Dean’s Emerging Scholars Research Awards
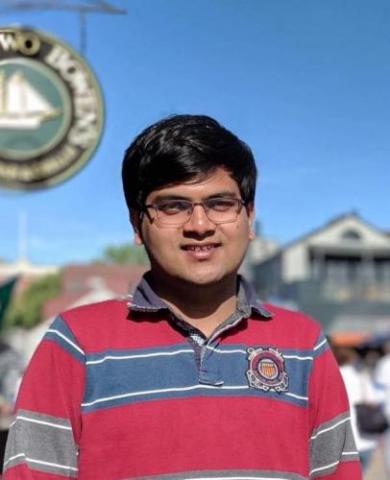
Yale Astronomy Ph.D. Candidate, Aritra Ghosh, has been selected to receive one of ten competitive Dean’s Emerging Scholars Research Awards. This award is given out each year by the Office of the Provost and the Graduate School of Arts and Sciences to recognize outstanding academic promise and achievement and significant contributions to diversity-related initiatives on campus.
Aritra Ghosh is a 5th year Ph.D. candidate working in Prof. Meg Urry’s group. Aritra uses the latest advances in machine learning combined with traditional techniques to study the evolution of galaxies and how that process is affected by supermassive black holes that reside in them.
For almost a century, starting Edwin Hubble’s work in 1926, astronomers have tried to link galaxies’ morphology (shape) to the physical processes behind how galaxies form and evolve. The morphology of a galaxy has been shown to be related to various fundamental properties of a galaxy and its environment – the total amount of mass in a galaxy, the rate at which the galaxy forms stars, the presence of supermassive black holes in the galaxy, and a range of other physics.
The next generation of astronomical surveys will allow us to study the morphology of billions of galaxies at different redshifts (i.e., distances from the Earth) – this will be crucial to understanding the physics of galaxy formation and evolution. However, the traditional methods of obtaining morphological classification – visual inspection and fitting models to galaxy light profiles – are not scalable to the massive data volumes expected from these surveys.
To overcome this challenge, Aritra and an extended team of astrophysicists and computer scientists have developed two machine learning (ML) frameworks – GaMorNet and GaMPEN. GaMorNet provides qualitative classifications for the shape of galaxies (e.g., whether a galaxy looks like a disk or a bulge), and GaMPEN provides qualitative estimates for different morphological parameters (such as the radius) along with robust uncertainties. These allow them to perform morphological analysis at a fraction (1/100th) of the time needed by traditional techniques. They have also used a novel approach of first training their models on simulated galaxies and fine-tuning them on actual galaxy images. This has allowed them to overcome the requirement of a sizeable pre-classified training set (a usual hurdle of ML algorithms). They have already demonstrated the accuracy of their frameworks on different astronomical surveys and shown that they are not black-boxes but interpretable by using various visualization techniques.
The team is now applying their algorithms to two extensive surveys conducted using space and ground-based telescopes to analyze the morphology of millions of galaxies at different redshifts. Besides obtaining the morphology of a large previously unstudied swathe of galaxies, they are using this information to learn more about how our universe evolved to its current state. To do this, they are correlating the morphological information with the amount of X-Rays detected at the centers of these galaxies. The amount of X-Rays indicates how active the supermassive black holes (BHs) present at the centers of these galaxies are.
Over the last two decades, studies trying to understand the impact of BHs on the morphology of their host galaxies have led to conflicting results – primarily due to low number statistics and the use of a separate algorithm by each study. Thus, how exactly BHs affect the formation and evolution of galaxies has remained a mystery. By studying the morphology of an extensive sample using a single ML technique, Aritra hopes to shed light on this major outstanding question in modern astrophysics.